We recently surveyed nearly 700 AI practitioners and leaders worldwide to uncover the biggest hurdles AI teams face today. What emerged was a troubling pattern: nearly half (45%) of respondents lack confidence in their AI models.
Despite heavy investments in infrastructure, many teams are forced to rely on tools that fail to provide the observability and monitoring needed to ensure reliable, accurate results.
This gap leaves too many organizations unable to safely scale their AI or realize its full value.
This isn’t just a technical hurdle – it’s also a business one. Growing risks, tighter regulations, and stalled AI efforts have real consequences.
For AI leaders, the mandate is clear: close these gaps with smarter tools and frameworks to scale AI with confidence and maintain a competitive edge.
Why confidence is the top AI practitioner pain point
The challenge of building confidence in AI systems affects organizations of all sizes and experience levels, from those just beginning their AI journeys to those with established expertise.
Many practitioners feel stuck, as described by one ML Engineer in the Unmet AI Needs survey:
“We’re not up to the same standards other, larger companies are performing at. The reliability of our systems isn’t as good as a result. I wish we had more rigor around testing and security.”
This sentiment reflects a broader reality facing AI teams today. Gaps in confidence, observability, and monitoring present persistent pain points that hinder progress, including:
- Lack of trust in generative AI outputs quality. Teams struggle with tools that fail to catch hallucinations, inaccuracies, or irrelevant responses, leading to unreliable outputs.
- Limited ability to intervene in real-time. When models exhibit unexpected behavior in production, practitioners often lack effective tools to intervene or moderate quickly.
- Inefficient alerting systems. Current notification solutions are noisy, inflexible, and fail to elevate the most critical problems, delaying resolution.
- Insufficient visibility across environments. A lack of observability makes it difficult to track security vulnerabilities, spot accuracy gaps, or trace an issue to its source across AI workflows.
- Decline in model performance over time. Without proper monitoring and retraining strategies, predictive models in production gradually lose reliability, creating operational risk.
Even seasoned teams with robust resources are grappling with these issues, underscoring the significant gaps in existing AI infrastructure. To overcome these barriers, organizations – and their AI leaders – must focus on adopting stronger tools and processes that empower practitioners, instill confidence, and support the scalable growth of AI initiatives.
Why effective AI governance is critical for enterprise AI adoption
Confidence is the foundation for successful AI adoption, directly influencing ROI and scalability. Yet governance gaps like lack of information security, model documentation, and seamless observability can create a downward spiral that undermines progress, leading to a cascade of challenges.
When governance is weak, AI practitioners struggle to build and maintain accurate, reliable models. This undermines end-user trust, stalls adoption, and prevents AI from reaching critical mass.
Poorly governed AI models are prone to leaking sensitive information and falling victim to prompt injection attacks, where malicious inputs manipulate a model’s behavior. These vulnerabilities can result in regulatory fines and lasting reputational damage. In the case of consumer-facing models, solutions can quickly erode customer trust with inaccurate or unreliable responses.
Ultimately, such consequences can turn AI from a growth-driving asset into a liability that undermines business goals.
Confidence issues are uniquely difficult to overcome because they can only be solved by highly customizable and integrated solutions, rather than a single tool. Hyperscalers and open source tools typically offer piecemeal solutions that address aspects of confidence, observability, and monitoring, but that approach shifts the burden to already overwhelmed and frustrated AI practitioners.
Closing the confidence gap requires dedicated investments in holistic solutions; tools that alleviate the burden on practitioners while enabling organizations to scale AI responsibly.
Improving confidence starts with removing the burden on AI practitioners through effective tooling. Auditing AI infrastructure often uncovers gaps and inefficiencies that are negatively impacting confidence and waste budgets.
Specifically, here are some things AI leaders and their teams should look out for:
- Duplicative tools. Overlapping tools waste resources and complicate learning.
- Disconnected tools. Complex setups force time-consuming integrations without solving governance gaps.
- Shadow AI infrastructure. Improvised tech stacks lead to inconsistent processes and security gaps.
- Tools in closed ecosystems: Tools that lock you into walled gardens or require teams to change their workflows. Observability and governance should integrate seamlessly with existing tools and workflows to avoid friction and enable adoption.
Understanding current infrastructure helps identify gaps and informs investment plans. Effective AI platforms should focus on:
- Observability. Real-time monitoring and analysis and full traceability to quickly identify vulnerabilities and address issues.
- Security. Enforcing centralized control and ensuring AI systems consistently meet security standards.
- Compliance. Guards, tests, and documentation to ensure AI systems comply with regulations, policies, and industry standards.
By focusing on governance capabilities, organizations can make smarter AI investments, enhancing focus on improving model performance and reliability, and increasing confidence and adoption.
Global Credit: AI governance in action
When Global Credit wanted to reach a wider range of potential customers, they needed a swift, accurate risk assessment for loan applications. Led by Chief Risk Officer and Chief Data Officer Tamara Harutyunyan, they turned to AI.
In just eight weeks, they developed and delivered a model that allowed the lender to increase their loan acceptance rate — and revenue — without increasing business risk.
This speed was a critical competitive advantage, but Harutyunyan also valued the comprehensive AI governance that offered real-time data drift insights, allowing timely model updates that enabled her team to maintain reliability and revenue goals.
Governance was crucial for delivering a model that expanded Global Credit’s customer base without exposing the business to unnecessary risk. Their AI team can monitor and explain model behavior quickly, and is ready to intervene if needed.
The AI platform also provided essential visibility and explainability behind models, ensuring compliance with regulatory standards. This gave Harutyunyan’s team confidence in their model and enabled them to explore new use cases while staying compliant, even amid regulatory changes.
Improving AI maturity and confidence
AI maturity reflects an organization’s ability to consistently develop, deliver, and govern predictive and generative AI models. While confidence issues affect all maturity levels, enhancing AI maturity requires investing in platforms that close the confidence gap.
Critical features include:
- Centralized model management for predictive and generative AI across all environments.
- Real-time intervention and moderation to protect against vulnerabilities like PII leakage, prompt injection attacks, and inaccurate responses.
- Customizable guard models and techniques to establish safeguards for specific business needs, regulations, and risks.
- Security shield for external models to secure and govern all models, including LLMs.
- Integration into CI/CD pipelines or MLFlow registry to streamline and standardize testing and validation.
- Real-time monitoring with automated governance policies and custom metrics that ensure robust protection.
- Pre-deployment AI red-teaming for jailbreaks, bias, inaccuracies, toxicity, and compliance issues to prevent issues before a model is deployed to production.
- Performance management of AI in production to prevent project failure, addressing the 90% failure rate due to poor productization.
These features help standardize observability, monitoring, and real-time performance management, enabling scalable AI that your users trust.
A pathway to AI governance starts with smarter AI infrastructure
The confidence gap plagues 45% of teams, but that doesn’t mean they’re impossible to overcome.
Understanding the full breadth of capabilities – observability, monitoring, and real-time performance management – can help AI leaders assess their current infrastructure for critical gaps and make smarter investments in new tooling.
When AI infrastructure actually addresses practitioner pain, businesses can confidently deliver predictive and generative AI solutions that help them meet their goals.
Download the Unmet AI Needs Survey for a complete view into the most common AI practitioner pain points and start building your smarter AI investment strategy.
About the author
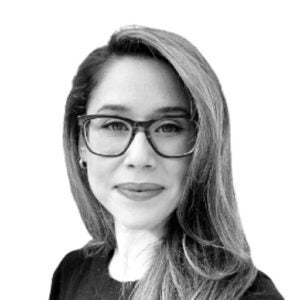
Lisa Aguilar is VP of Product Marketing and Field CTOs at DataRobot, where she is responsible for building and executing the go-to-market strategy for their AI-driven forecasting product line. As part of her role, she partners closely with the product management and development teams to identify key solutions that can address the needs of retailers, manufacturers, and financial service providers with AI. Prior to DataRobot, Lisa was at ThoughtSpot, the leader in Search and AI-Driven Analytics.